15%
Decreased in Stock Level for the Same Revenue
30%
Cutting of Truck Count in the Same Transportation Workload
20%
Saving in Supply Chain Cost
About the Client
Our customer has a supermarket chain in Vietnam with over 1,000 stores nationwide. It sells various products, including food, apparel, electronics, and home furnishings.
Solution
We collected and processed data using Microsoft services to improve supply chain & sales operations. For the supply chain, the system generated PO suggestions with ML to optimize routes & delivery times. For sales & marketing, we analyzed customer behaviors to generate promotion recommendations.
Overview
Industry: Retail
Technology: Azure Synapse Analytic, Power BI, Azure Analytic, Azure Data Lake Gen 2
Service Domain: Cloud, Data Factory, Data Lake, BI and Analytics
Background and Challenge Story
At that time, our client needed to improve their supply chain efficiency and sales activities and implement the best practice of data platforms to adapt to market needs. As our client’s ordering activities mostly relied on the experience of supermarket and warehouse managers, it led to high ordering and inventory costs. Besides, marketing promotions only focused on pushing slow-selling goods and campaigns from suppliers, which is unbeneficial to the business revenue. Their operational system was outdated, frequently overloaded, and poor performed, especially during the data extraction period for reporting purposes.
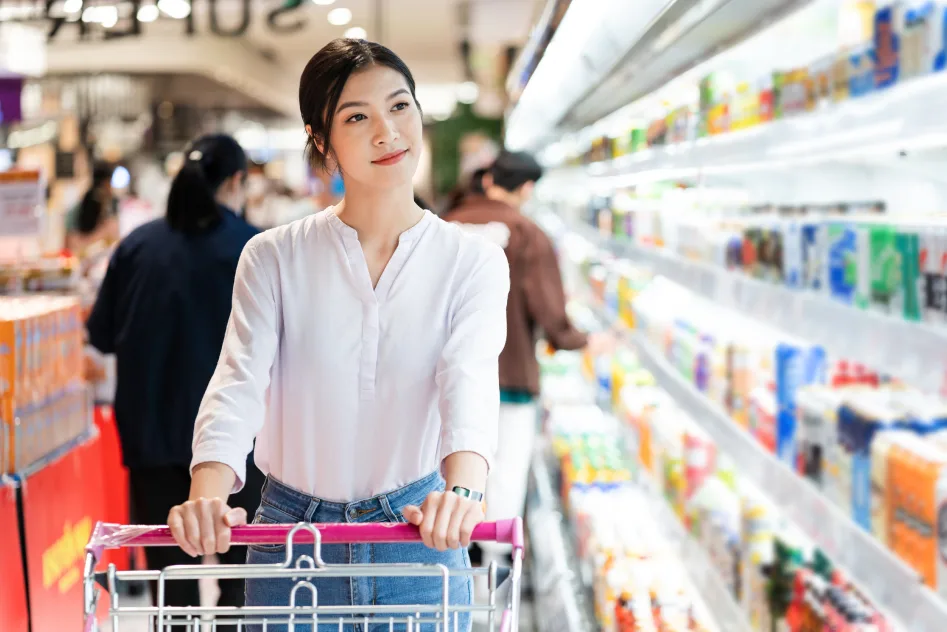
CMC’s Approach
We implemented the project by collecting and consolidating the processing of enormous data volume from the import, export, and selling transactions of 30+ supermarkets & 7 warehouses using Microsoft services. Subsequently, we utilized data to optimize the supply chain and sales operations.
For the supply chain: Based on the current stock level, sell-in, and sell-out history, the system forecasted and automatically generated PO suggestions with detailed goods and quantities. These activities shortened the ordering process and optimized inventory costs, therefore reducing supply chain costs. We also applied the machine learning (ML) model to optimize the route and delivery in-time points to save transportation costs.
For sales and marketing operations: Our data system analyzed customer behaviors and automatically generated promotion recommendations. These programs have proven effective in increasing customers’ transactions at the supermarket and creating larger purchases. The methodologies applied include Cohort analysis, RFM analysis, and some recommended ML models..
Impact
The successful application of machine learning in many activities: PO suggestion, optimizing the route and on-time delivery point, individual promotion recommendation, highly available and stable operational system, centralizing monitoring and cost management has led to the following results:
• 15% Decrease in stock level while maintaining the same revenue
• 30% Cutting of truck count for the same transportation workload
• 10% Increase in Bill count and 20% increase in cart value
• 20% Increase in customer return rate
• 20% Supply chain cost savings